Graph Based Association Analysis for the Business to Business Domain
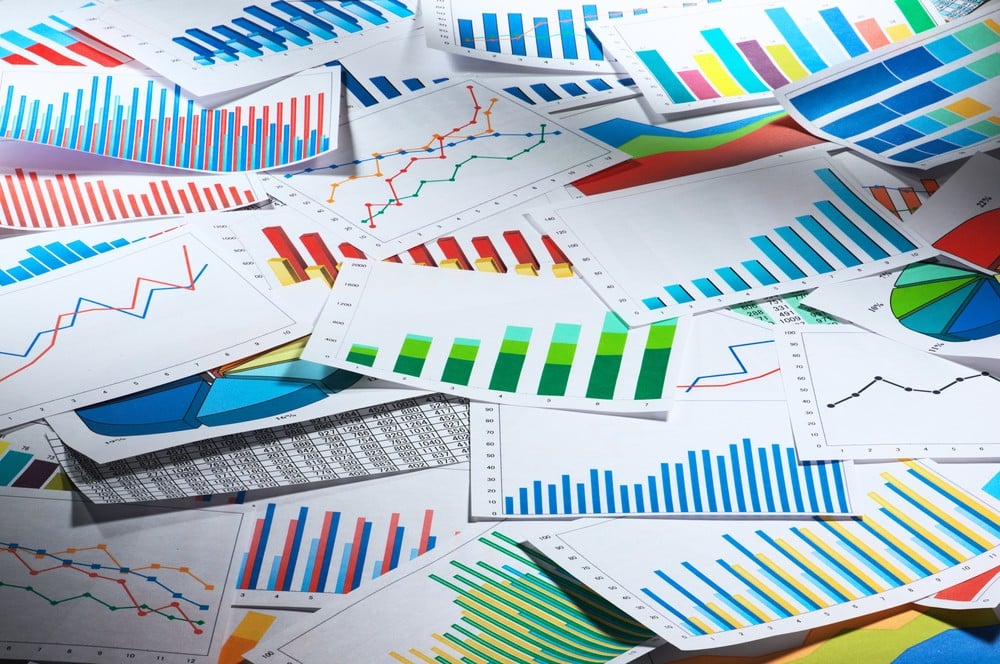
In this paper, we discuss some of the problems in B2B (Business to Business) domain and discuss how graph based association analysis can solve those with a focus on interactions among companies, though we also consider social network within an organization.
Data Mining tools may no longer be sufficient or even relevant to handle explosion in data types. Graphs are a great visualization aid. Conversations today have become digitized, and so have the tacit components; but are still fuzzy.
Here are B2B use cases that can be solved with Graphs: a) predict which clients could churn, b) detect relationships between businesses breaking away, c) predict which market will suffer next, such that the insights from it can help in generating better simulations, d) find key targets for acquisition, and e) content based link prediction for patent marketing.
We explain algorithms on ranking using graphs and community detection in intersecting communities. We consider graph learning problems where the goal is to rank the objects relative to one another. We mention how B2B domain graph structure differs from that of Consumer segment. We mention support vector machines (SVM) because a) IBM Patent [7] used it for link prediction, and b) we got good results from our data on order fallout management. Our focus is to build use cases for association analysis in the B2B domain using graph theory, for which we can use some of the algorithms mentioned by [13] and [17] because solving the problem with large graphs can become an NP complete problem, and [13] and [17] solve the NP complete problem. In the end, we construct a call graph as a motivation for this work.
General Information